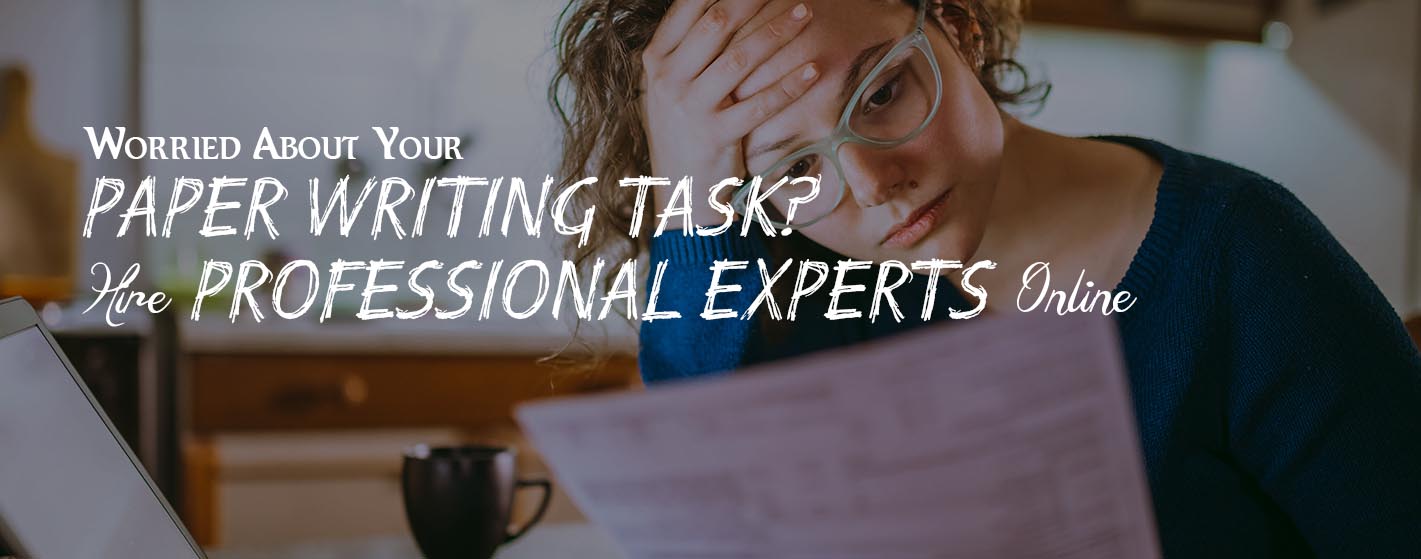
Converting Likert scale responses to fuzzy set calibrations is a crucial step in many research studies and decision-making processes, especially in fields where uncertainty plays a significant role. Fuzzy logic offers a flexible framework to be used in your custom dissertation writing for handling imprecise or vague information, allowing for more nuanced analysis and interpretation.
Fuzzy logic is a mathematical framework that deals with uncertainty by allowing values to range between 0 and 1, representing degrees of truth or membership. Unlike classical logic, which operates with crisp (binary) values, fuzzy logic enables the representation of vague or ambiguous information more effectively. By using fuzzy sets and membership functions, fuzzy logic provides a way to model and reason with imprecision.
Membership functions are essential components of fuzzy sets to be used in A Plus custom dissertation writing, defining the degree of membership of an element in a set. When converting Likert scale responses to fuzzy set calibrations, it's crucial to define appropriate membership functions that capture the essence of the Likert scale levels. These functions can take various shapes, such as triangular, trapezoidal, or Gaussian, depending on the characteristics of the data and the context of the personalized dissertation writing.
Linguistic terms are descriptors used to label the levels of the Likert scale, such as "Strongly Disagree," "Disagree," "Neutral," "Agree," and "Strongly Agree." These terms serve as reference points for mapping Likert scale values to corresponding fuzzy membership degrees. By associating each linguistic term with a specific range of membership degrees, we can create a bridge between the ordinal Likert scale and the fuzzy logic framework.
The next step involves assigning membership degrees to each linguistic term based on its position on the Likert scale. A professional expert at cheap custom dissertation writing service can guide you in this regard. For example, if the Likert scale ranges from 1 to 5, we might assign membership degrees of 0, 0.25, 0.5, 0.75, and 1 to the linguistic terms "Strongly Disagree," "Disagree," "Neutral," "Agree," and "Strongly Agree," respectively. These membership degrees reflect the skilled dissertation writer about the degree of agreement or disagreement with the statement being evaluated.
The shape of the membership functions determines how membership degrees are distributed within the defined ranges. Triangular membership functions are commonly used due to their simplicity, but other shapes such as trapezoidal or Gaussian can also be employed based on the characteristics of the data and the desired interpretation. Best dissertation writing service can assist you with experimentation with different shapes may be necessary to find the most suitable representation for the given context.
When converting Likert scale responses to fuzzy set calibrations, a university dissertation writer must decide whether to interpolate or extrapolate membership degrees for values between and beyond the discrete Likert scale points. Interpolation involves estimating membership degrees within the defined Likert scale range, while extrapolation extends this estimation beyond the defined range. The choice between interpolation and extrapolation depends on the specific requirements of the analysis and the availability of data.
Validation of the fuzzy set calibrations is essential to ensure their accuracy and reliability. This can be done through testing the fuzzy logic model against known data or by seeking feedback from domain experts. If inconsistencies or inaccuracies are identified during validation, refinements to the membership functions or the mapping process may be necessary to improve the model's performance.
Documenting the conversion process may need you to buy dissertation help from cheap writing deal is crucial for transparency and reproducibility. This documentation should include the definitions of linguistic terms, the rationale behind the assignment of membership degrees, the shapes of the membership functions used, and any validation or refinement steps undertaken. Clear documentation enables other researchers to understand and replicate the process, enhancing the credibility of the study's findings.
Contextual factors such as the domain of the study, the characteristics of the data, and the specific objectives of the analysis should be taken into account throughout the conversion process. The interpretation of fuzzy set calibrations may vary depending on these factors, so it's essential to consider them carefully when making decisions about membership functions and mapping strategies.
If you're unsure about certain aspects of the conversion process or the interpretation of fuzzy set calibrations, it can be helpful to consult with experts in fuzzy logic or the relevant domain. Experts can provide valuable insights and guidance, helping to ensure the validity and effectiveness of the fuzzy logic model.
Converting Likert scale responses to fuzzy set calibrations is a nuanced process that requires careful consideration of various factors, including membership functions, linguistic terms, interpolation/extrapolation methods, validation procedures, and contextual factors. By following the steps outlined in this guide and taking these considerations into account, researchers and decision-makers can effectively leverage fuzzy logic to handle uncertainty and make more informed decisions in a wide range of applications.